- Neville said on March 3 that Liverpool and Arsenal had to beat Manchester City
- They didn’t achieve it and now Pep Guardiola’s men have control in the race for the title
- IAN LADYMAN and CHRIS SUTTON weigh in on the Premier League Player of the Year – Listen to the everything is beginning podcast
A Premier League title race prediction made by Gary Neville last month could well prove eerily accurate as Manchester City took the lead entering the final stretch.
Speaking after City beat Manchester United 3-1 in the derby on March 3, Neville said on Sky Sports that Liverpool and Arsenal had to beat Pep Guardiola’s team to have a chance.
A week later, City went to Anfield and emerged with a 1-1 draw despite the best efforts of Jurgen Klopp’s men.
On March 31, Arsenal arrived at the Etihad Stadium and the title clash ended in a goalless draw.
Having failed to beat City, both Liverpool and Arsenal now feel the intense pressure of the title race.
Gary Neville’s very accurate prediction of how the Premier League title race will unfold has resurfaced after Manchester City capitalized on mistakes by Arsenal and Liverpool.
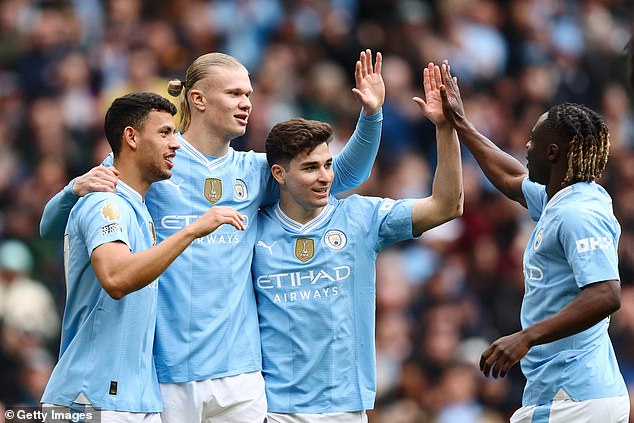
The title looks set to go to Manchester City after taking the lead over the weekend.
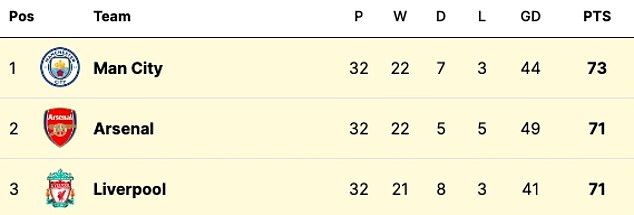
City remained top after Liverpool and Arsenal suffered damaging defeats on Sunday.
Your browser does not support iframes.
After Guardiola’s team thrashed Luton 5-1 on Saturday, Liverpool lost 1-0 to Crystal Palace and Arsenal lost 2-0 to Aston Villa in major blows to their title aspirations.
It left City two points clear at the top of the table with six games left to play and imagining their chances of winning four titles in a row.
The clip showing former Manchester United defender Neville predicting precisely this emerged on Sunday night after both City’s title rivals lost.
In it, Neville said: “I really think Liverpool and Arsenal must think they can beat Manchester City.”
“They have to think this way to create this monumental shift in the belief that City are going to win the league, it’s their time of year, they have experience, they’ve won three in a row.”
“I always think that when we lose titles, whether with Chelsea or [Carlo] Ancelotti, or [Sylvain] Wiltord at Old Trafford, or [Marc] Overmars scored at Old Trafford, the team that won came to Old Trafford and won.
“They didn’t just come to Old Trafford, draw and get a point and do well, they came and surprised us.”
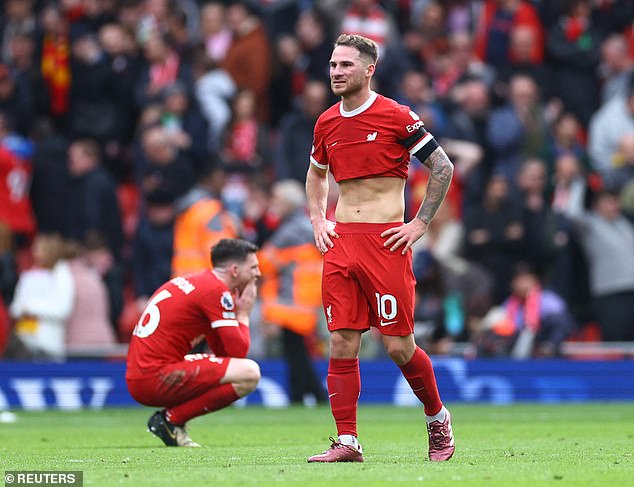
This came after Liverpool lost 1-0 at home to Crystal Palace, in a major blow to their title ambitions.
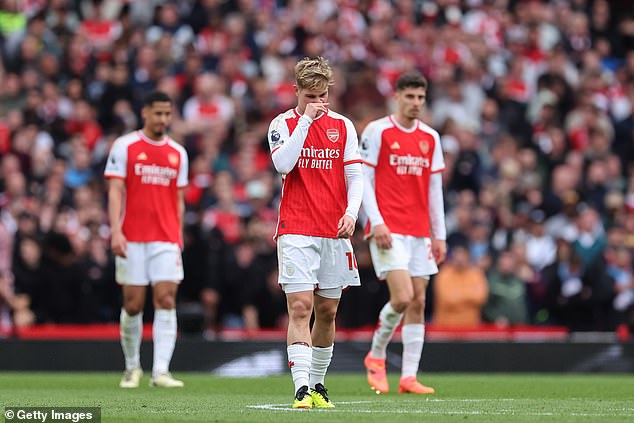
Arsenal lost 2-0 at home to Aston Villa and their chances of winning the title took a huge hit.
“I think that’s what Liverpool and Arsenal have to do to take the faith out of this Pep Guardiola team because they’ll think it’s sinister at the moment.”
That has not happened and now City look favorites to win again given their vast experience in winning titles in recent seasons.
Next weekend, Guardiola’s team will face Chelsea in the FA Cup semi-final at Wembley. Arsenal and Liverpool are in league action, visiting Wolves and Fulham respectively.